Optimizing Wastewater Asset Management through Predictive Analytics
Freya Systems developed an algorithm to reduce operational costs in wastewater treatment.
Need
Background
Approach
The Objective
The Approach
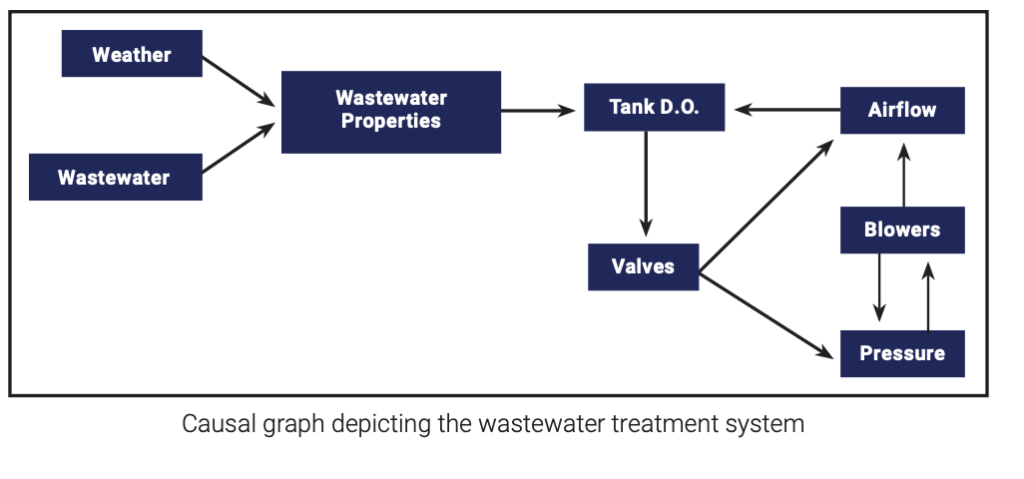
Results
Among all the days in which all blowers were used concurrently, the algorithm successfully predicted the occurrence beforehand every time.
The Result
Among all the days in which all blowers were used concurrently, the algorithm successfully predicted the occurrence beforehand every time.
Following this monitoring period, the client deemed the algorithm a success and is actively working to fully integrate the algorithm’s predictions into the automated treatment process.
The algorithm demonstrated strong initial performance, leading to on-site implementation and multi-month monitoring.
Among all days where the algorithm predicted full blower usage, blowers were indeed used concurrently on approximately two out of three days.
Whenever all blowers did run concurrently, the algorithm successfully predicted the occurrence every time.
Conclusion
After six months of real-world operation, Freya Systems analyzed the post-implementation data and found that the algorithm could reduce energy consumption on blower functions by 6.4%. This is especially significant given that blower systems account for roughly 40% of overall energy use in wastewater processes. By integrating predictive analytics into the automated workflow, the facility could save on operational costs and also prolong equipment life—an important step toward enhancing both sustainability and cost efficiency in modern wastewater treatment.
Freya Systems remains committed to helping utilities harness the power of advanced analytics, ensuring that every insight gained delivers measurable value and supports the long-term health of critical infrastructure.